Better Decision Support for
Better Urban Governance
BeDS for BUG
Better Decision Support for
Better Urban Governance
BeDS for BUG
Mejor Apoyo a la Decision
para
Mejor Gobernanza Urbana
Brasília
São Paulo
Suzano
Cairo
Guayaquil
Chennai
Guadalajara
Coimbra
Marinha Grande
Manchester
Boston
Brasil
Egipto
Ecuador
India
Mexico
Portugal
Reino Unido
EEUU
GANs for Synthetic Cities and Regions
Synthetic Urban
Synthetic cities to support the development of advanced urban models
In today’s technologically-driven world, data has become a vital asset that drives growth and innovation in multiple domains of human activity. It provides insights into the complex dynamics of society, economy, and government, empowering policymakers, stakeholders (and even citizens) to make educated decisions (Kushwaha et al., 2021).
Census data differs from other sorts of data in that it gives a comprehensive and detailed review of various aspects of a country every ten years. It is conducted periodically with the purpose of enumerating the entire population of a country and collecting detailed information about their demographic, social, and economic characteristics. This data plays a crucial role in comprehending population dynamics, migration patterns, employment trends, and urban growth, and it informs policy making and resource allocation (Wesolowski et al., 2013; O’Hare, 2019). Collecting such socio-economic data poses significant challenges and can be a costly activity. At the same time, using this data to develop and test models to inform decision-making and policy design and testing for urban issues is key for developing accurate models that effectively assist these processes..
The use of incomplete, insufficient or even obsolete census data for decision-making can have a detrimental influence on the development of urban simulation models of all sorts (e.g. land use, transport or econometric models) for policy design and testing.
Synthetic data serves as a solution to better develop urban models. The potential of synthetic census data to enhance the development and testing of data-driven urban models has great potential, as it creates generalizable datasets free of potential location-specific bias, errors or data quality issues. It gives freedom to model developers to conceptualise and implement modelling concepts focusing on validation rather than immediate calibration. These models can be tested against more unconstrained assumptions, allowing model users to develop a deeper understanding of urban phenomena. Which in turn may empower stakeholders and policymakers with the ability to assess policy impacts prior to implementation.
While Generative Adversarial Networks (GANs) have received extensive research attention, their primary focus has been on generating synthetic images rather than alphanumeric data. Attempts have been made to generate synthetic tabular data through GANs, yet limited, particularly with regard to census data. This limitation highlights the novelty of our study, which is aimed towards the generation of synthetic census data.
Through this study, we have developed a GAN capable of producing synthetic census statistics comprising key demographic, economic, deprivation, and transportation variables. The state-of-the-art DATGAN architecture served as the foundational framework for our model (Lederrey et al., 2022). Additionally, we created synthetic spatial boundaries corresponding to the generated census data at various spatial levels.
We developed a tool using a user-friendly Jupyter notebook that enables the simulation of the entire workflow. This tool facilitates the generation of synthetic census statistics and spatial boundaries at various spatial scales through user-defined inputs.
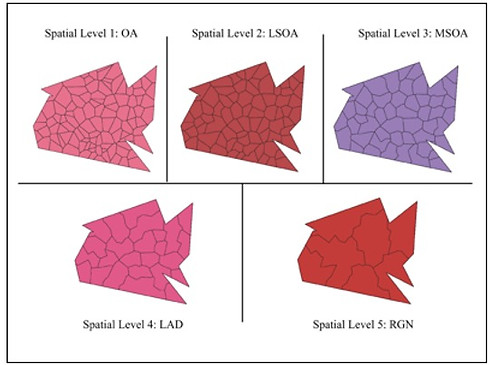
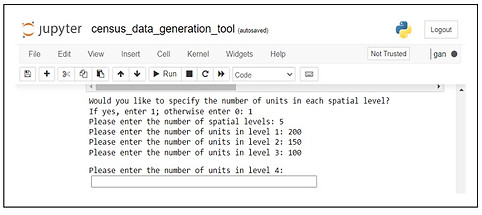
Figure 2: Census data generation tool
Figure 1: Synthetic spatial geometries at five spatial levels
References
Kushwaha, A. K., Kar, A. K. and Dwivedi, Y. K. (2021). Applications of big data in emerging manage- ment disciplines: A literature review using text mining, International Journal of Information Man- agement Data Insights 1(2): 100017. Available at: https://doi.org/10.1016/j.jjimei.2021. 100017
O’Hare, W. P. (2019). The Importance of Census Accuracy: Uses of Census Data, in W. P. O’Hare (ed.), Differential Undercounts in the U.S. Census: Who is Missed?, Springer International Publishing, Cham, pp. 13–24. Available at: https://doi.org/10.1007/978-3-030-10973-8_2
Wesolowski, A., Buckee, C. O., Pindolia, D. K., Eagle, N., Smith, D. L., Garcia, A. J. and Tatem, A. J. (2013). The Use of Census Migration Data to Approximate Human Movement Patterns across Temporal Scales, PLOS ONE 8(1): e52971. Publisher: Public Library of Science. Available at: https://doi.org/10.1371/journal.pone.0052971
Lederrey, G., Hillel, T. and Bierlaire, M. (2022). DATGAN: Integrating expert knowledge into deep learning for synthetic tabular data. Available at: http://arxiv.org/abs/2203.03489
Outputs [P] [C] [O] [E]
No outputs have been produced yet for this project.
Project partners
This project has been developed under a MSc Data Science Urban Analytics dissertation.
Research Team
The project is lead by Miss Mariam Jamilah (MSc Studnet) with conceptualisation and supervision by Dr Nuno Pinto.